- Jan 10, 2021
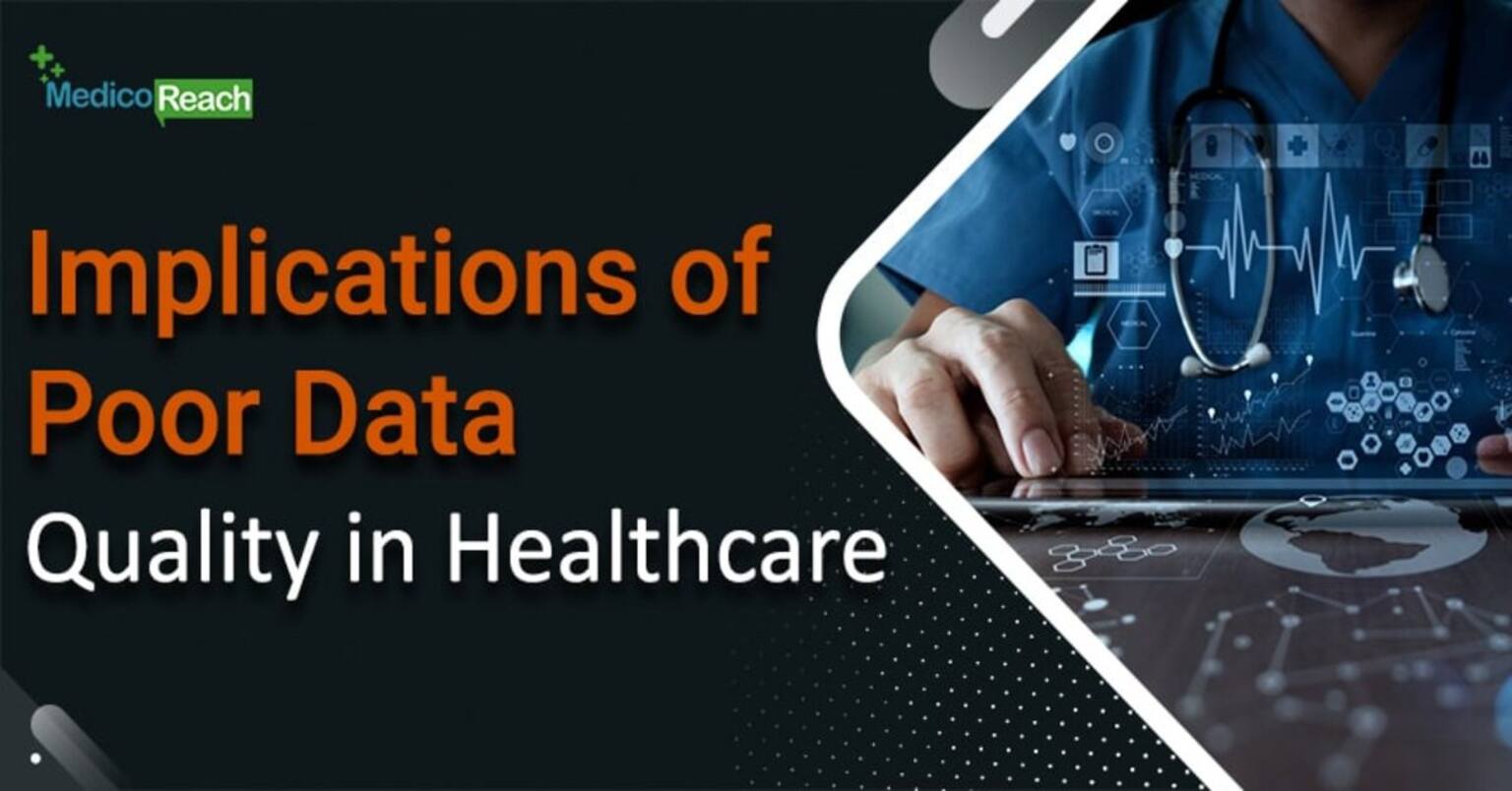
In today’s evolving healthcare landscape, organizations require unprecedented reliability and clarity of their data for improving analytics and reporting, prevent duplication of services, facilitate smooth billing processes, and increase patient safety.
Maintaining proper data quality is critical for all industries, especially for risk-averse sectors like healthcare. This is a field where any information obtained from data could literally make all the difference between both life and death.
The impact of poor data quality in healthcare is a serious matter. In fact, Gartner’s research suggests that poor data quality costs businesses about $9.7-14.2 million each year. While these figures reflect every industry type, it’s still important to know.
In this blog, we’ll be covering the implications of bad data in healthcare to help you understand the seriousness of the matter. This way, you can work towards ensuring that your healthcare database is always reliable.
4 Significant Issues Resulting From Bad Data Quality
Though maintaining solid data quality might appear like a minor implementation or operational concern, it should be taken quite seriously by every stakeholder. In healthcare, data quality is incredibly important as you can see from the example below.
Poor quality data can often cause complications across an organization and this complication includes everything from the policy-level resolution to treatment quality. Bad quality data can cause some serious implications, they include:
Patient Mistreatment & frustration
If the healthcare database has improperly entered source data, one has to manually intervene and resolve any inaccuracies and/or discrepancies that might be present within the system.
The problem with this manual approach, however, is that it can sometimes result in delays and worse, even mistreatments. All of these will ultimately lead to poor patient experiences.
Employee Distrust in Data
In an organization where human intervention is prevalent, employees will start getting frustrated and lack trust in the data they’re given.
Managing and even just coping with poor data quality can have a big influence on employee morale. Healthcare workers who were employed to handle high-level tasks are very less likely to gain satisfaction from manual data cleanup. On the other hand, the frustration of working with inconsistent, inaccurate, or incomplete data makes the overall work more complex and less satisfying.
Aside from basic moral complications, this will cause healthcare staff to return to manual analysis and reporting. This will further increase the chances of errors and deter them from effectively collecting valuable data.
Less Productivity
“Quality data translates to higher facility performance”
Poor quality data inaccuracies can significantly lower productivity, cause inefficiencies, and boost operational costs. With regular manual intervention to rectify inaccurate data stopping or slowing jobs on otherwise automatable operations, employee efficiency is significantly affected. This can cause work backlogs and inconsistent procedures that might have been prevented with more systematic strategies.
When healthcare employees manage data complications manually, they sometimes choose to go for quick rectifications rather than solving the underlying problem, particularly if they need to meet their deadline. The issue with this is that it increases the possibility of human errors, which can cause further complications down the line.
Poor Decision Making
As healthcare management and policy-makers are increasingly dependent on large datasets for making smarter & more informed decisions, data inaccuracies can result in improper decisions. As a healthcare professional, you need good data so you can make the most appropriate decisions and strategies for your organization.
Since decision making is generally done using aggregated datasets, even minor inaccuracies in the data source can increase when combined with large datasets.
Harms Reputation
The harm done by poor quality data isn’t just limited within your company. Quality issues with healthcare data impact your patients as well. Billing errors, appointment or scheduling mistakes, etc., can all cause more frustration to your clients.
And though the impact of rectifying these complications will be felt by your customer service team, the main challenge will arise when those frustrated patients start pouring in their reviews.
Regardless of the industry, it’s crucial to manage customer satisfaction. This is because even a single bad review can harm the organization’s reputation. According to research, it takes forty good customer experiences for undoing the harm done by one negative feedback. Reviews generally play a major role in the decision-making process of potential patients.
So, What Causes Healthcare Poor Quality Data?
Poor data quality is not the outcome of a single flaw, rather it’s due to the culmination of a number of factors. Among many the many concerns, duplication remains the biggest one.
In fact, about 5-10% of records in a hospital’s EHR have duplicate inputs, according to research. That figure further increases to 20 percent for healthcare organizations that have different locations.
Duplication occurs due to multiple reasons, one of which includes spelling errors or other mistakes in patient data. Another reason for poor data quality is because it’s incomplete.
A record that has missing fields is basically useless. If a patient data fail to include things like allergies or preexisting conditions, incomplete data isn’t the only issue. It can even impact care.
Another significant cause of poor data is inaccuracies. This can arise if the data hasn’t been updated correctly or due to transposed numbers, misspelled names. Without accurate patient data, whether it’s insurance codes or contact information, it’s difficult to contact your customers and leverage your data for better insight and outcome.
How To Meet Healthcare Data Quality Goals?
To ensure high-quality data for the healthcare industry, entities must procure strong data cleansing solutions. Not only will it help in maintaining high-quality data, but it’ll also help healthcare organizations to meet regulatory goals.
Data cleaning refers to the process of identifying and rectifying inaccurate, corrupted or duplicate data. This data, particularly when it concerns healthcare, must not be incomplete, inaccurate, wrong, or unrecognizable to the processes and operations that use them. Data cleansing also detects duplicate information, which directly affects the efficiency and effectiveness of an organization. For healthcare entities to operate efficiently and make accurate decisions, they must engrain these processes and activities in their daily operations.
Final Say
While incredibly powerful, data can have serious negative implications if the information gathered, saved, and examined is incomplete and accurate.
Also, while complying with the requirements of HIPAA, PSQIA and HITECH might seem demanding, these regulations are required and healthcare professionals must ensure they’re fully compliant.
The best way to do this is by employing a solid data cleansing strategy that doesn’t only address these regulatory requirements but also ensures data privacy and accuracy. This will allow organizations to minimize the impact of poor data quality in healthcare.